找出分类器的最优参数
加载数据集并将其分为训练集合和测试集合.
In[1]:=
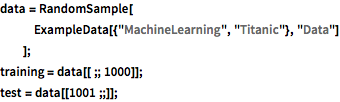
data = RandomSample[
ExampleData[{"MachineLearning", "Titanic"}, "Data"]
];
training = data[[;; 1000]];
test = data[[1001 ;;]];
定义一个计算分类器性能的函数,它可以作为其(超)参数的函数.
In[2]:=
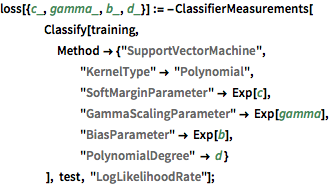
loss[{c_, gamma_, b_, d_}] := -ClassifierMeasurements[
Classify[training,
Method -> {"SupportVectorMachine",
"KernelType" -> "Polynomial",
"SoftMarginParameter" -> Exp[c],
"GammaScalingParameter" -> Exp[gamma],
"BiasParameter" -> Exp[b],
"PolynomialDegree" -> d }
], test, "LogLikelihoodRate"];
定义参数的可能值.
In[3]:=
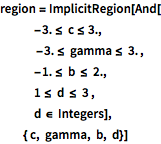
region = ImplicitRegion[And[
-3. <= c <= 3.,
-3. <= gamma <= 3. ,
-1. <= b <= 2.,
1 <= d <= 3 ,
d \[Element] Integers],
{ c, gamma, b, d}]
Out[3]=

查找参数的恰当数据.
In[4]:=

bmo = BayesianMinimization[loss, region]
Out[4]=

In[5]:=

bmo["MinimumConfiguration"]
Out[5]=

用这些参数培训分类器.
In[6]:=
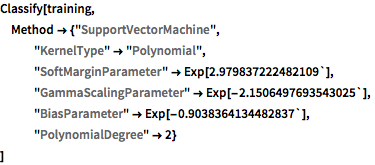
Classify[training,
Method -> {"SupportVectorMachine",
"KernelType" -> "Polynomial",
"SoftMarginParameter" -> Exp[2.979837222482109`],
"GammaScalingParameter" -> Exp[-2.1506497693543025`],
"BiasParameter" -> Exp[-0.9038364134482837`],
"PolynomialDegree" -> 2}
]
Out[6]=
