분류자의 최적 파라미터 찾기
데이터 집합을 로드하고 훈련 집합과 검증 집합으로 분할합니다.
In[1]:=
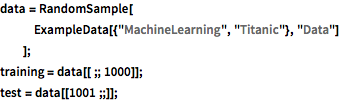
data = RandomSample[
ExampleData[{"MachineLearning", "Titanic"}, "Data"]
];
training = data[[;; 1000]];
test = data[[1001 ;;]];
분류자의 성능을 그 (하이퍼) 파라미터의 함수로 계산하는 함수를 정의합니다.
In[2]:=
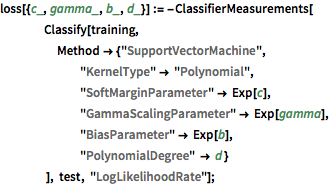
loss[{c_, gamma_, b_, d_}] := -ClassifierMeasurements[
Classify[training,
Method -> {"SupportVectorMachine",
"KernelType" -> "Polynomial",
"SoftMarginParameter" -> Exp[c],
"GammaScalingParameter" -> Exp[gamma],
"BiasParameter" -> Exp[b],
"PolynomialDegree" -> d }
], test, "LogLikelihoodRate"];
매개 변수의 가능한 값을 정의합니다.
In[3]:=
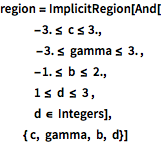
region = ImplicitRegion[And[
-3. <= c <= 3.,
-3. <= gamma <= 3. ,
-1. <= b <= 2.,
1 <= d <= 3 ,
d \[Element] Integers],
{ c, gamma, b, d}]
Out[3]=

매개 변수의 적절한 집합을 검색합니다.
In[4]:=

bmo = BayesianMinimization[loss, region]
Out[4]=

In[5]:=

bmo["MinimumConfiguration"]
Out[5]=

구해진 파라미터로 분류자를 훈련합니다.
In[6]:=
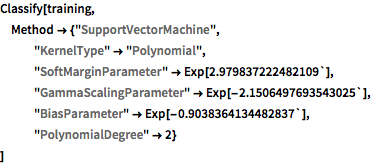
Classify[training,
Method -> {"SupportVectorMachine",
"KernelType" -> "Polynomial",
"SoftMarginParameter" -> Exp[2.979837222482109`],
"GammaScalingParameter" -> Exp[-2.1506497693543025`],
"BiasParameter" -> Exp[-0.9038364134482837`],
"PolynomialDegree" -> 2}
]
Out[6]=
